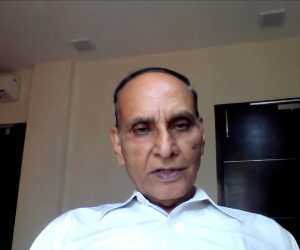
The Managing Director of InnovatioCuris Foundation of Healthcare & Excellence Dr. V.K Singh commenced the meeting with a brief introduction of IC InnovatorCLUB and the objective of the present session based on ‘What it takes to do real world AI: lessons from deployment’.
He divulged the present dilemma of relying on AI for every medical issue without any medical assistance from employees, the usage of telemedicine in India following the outbreak of the pandemic and also cited a number of Artificial Intelligence (AI) applications in the medical field. Further he mentioned several legal and ethical challenges surrounding AI, advising that we employ technology as a supplement to our efforts. Dr. Singh greeted the panelists and attendees of the session.
Dr. Cherian, Ms. Shraddha, and Mr. Rohit Ghosh, who joined remotely, were welcomed by Mr. Sachin Gaur, Executive Editor of InnoHEALTH magazine. He emphasized the importance of using AI in the medical industry with a brief overview of the meeting’s agenda and the flow of the session. The questions planned to be asked to the experts were in the realm of comprehension, such as what it takes to make an AI product successful in a clinical setting? From a technical standpoint as well as in terms of the actual obstacles and challenges they confront. Participants in the meeting are more likely to obtain insights and learn some crucial lessons if they are aiming to create a business.
Mr. Gaur welcomed the first panelist for this club meeting Mr. Rohit Ghosh who is the founding member and Chief strategy officer of Qure.ai.

Mr. Rohit discussed the difficulties they confront on the ground while installing AI. He added that Qure.AI has deployed AI in approximately 50 countries and 500 hospitals in the United States, the United Kingdom, Europe, Africa, Southeast Asia, and Asia. For smooth running of the installation, he devised a plan to comprehend some of the problems and lessons learned in due course and those he will be sharing during the session.
He initiated his questionnaire session with the first question on AI, “Do you need to enlarge the data sets for training?” “Could you explain the difficulties here”? He reciprocated that indeed the datasets for training are the most important item for any AI company. His company Qure.Ai has almost finished processing 4.2 million photos for a chest x-ray algorithm they developed. He underlined the need of using data sets for training, as this leads to accuracy. Although delta improvement necessitates a large amount of data where any amount of data counts.
In response to the second question, “How can we measure completeness of data, representation of groups, and other such things?” He explained that complete data is a theoretical concept where fluctuations such as regional, disease, and seasonal variations, are data sets that should be addressed more. He underlined the hardship to track down all of the data.
His next question was, “How objective are the ground truths of your training data sets, and what can you do to improve the quality objective of ground truth?” In response, Mr. Rohit stated that in AI, you must have an objective function, however in real life or reports, ground truths are not always as objective, such as when radiologists do not always take complete background of the case. So, the need to train any algorithm becomes important. Now, to improve their ground regularity quality, they’ve standardised ground truthing techniques, such as having a panel of radiologists review reports instead of just one. Another thing they have implemented is to construct a complete NLP ( National Language propository) terms that they use to represent such findings. Therefore it uses multiple reads instead of one to get the objective that a person normally gets from physicians.
“Are the outcomes the system gives explainable and interpretable to clinicians?” comes the next inquiry. Do you have a way to visualise and explain them in a more user-friendly interface or report”? According to Mr. Rohit, explainability is at the heart of machine learning and AI research at the moment, but in his interactions with physicians and radiologists, it is a minor problem because clinicians are already familiar with AI medical imaging.
The next question is what happens when AI and physicians disagree. Is it true that they provide feedback? He justified the query by explaining that there are times when AI and physicians disagree, but just because one result differs from the other does not mean the AI is erroneous. So they have a discordance meeting to discuss the cases that are discordant. Then it’s assessed by a panel, which gathers any discrepancies and trains the AI to release future versions.
The next topic was how to provide feedback on your system’s performance in a clinical situation. The discordance meeting has already been explained by him and there is also post-market surveillance alongwith a FDA regulatory approval for the algorithms. A subset of everyday assessments is also examined by a panel in order to determine whether AI is making the correct decisions. Qure reads exam samples and then rereads them. AI is just used to ensure that the quality is up to par on a daily basis.
“Does Deployment Change Care Pathways?” was the next question in the discussion. Is there a way to retrofit or intervene? In response, Mr Ghosh elucidated that retrofitting and intervention are both possible as it alters care patterns in some regions. Qure.AI has been able to make a difference since receiving WHO approval for TB diagnosis. The entire TB diagnosis takes one hour.
Finally, what value does your technology add to the healthcare process, such as improving the quality of clinical decision-making systems, automating manual processes, or something else? What do you do to build consensus on the impact? In your perspective, clinicians perceive a gain to the extent you foresee, so what do you do to build consensus on the impact? They’re basically increasing patient outcomes, according to Mr.Ghosh.
At Qure.ai, one of the use cases is to reduce work burden and manual labour. Radiologists’ turnaround time should be reduced so that reports may be produced more quickly and accurately. Early detection of severe disease and prompt treatment are essential.
In AI, there is a lot of agreement. There is a lot of maturity in the ecosystem right now. Rohit’s part of the meeting came to an end with that.
What value does your technology add to the healthcare process, such as improving the quality of clinical decision-making systems, automating manual processes, or something else? What do you do to build consensus on the impact?.
Sachin Gaur moderating the session welcomed the next panelist Dr. Cherian, Co-founder at Synapsica

Dr. Cherian introduced himself and gave an overview of Synapsica’s work. In terms of the data sets, he and Mr. Ghosh had different viewpoints. He told us that they have enough data and are working to extend their data sets so that they can build more features and capabilities in AI using the tools they already have. He noted that data preparation, objective ground truthing of data cleansing, and knowing how it will impact your AI system not just in terms of money but also in terms of time are all expensive inputs into the system so it is critical to maintain a sense of equilibrium. From a medical standpoint, adding additional data does not necessarily imply that the AI’s output will improve. You can construct more accurate algorithms by using updated algorithms and technological advances that can be used for learning from more data sets. The output is influenced by the quality of the algorithms.
Dr. Cherian agreed with Mr Ghosh that there is no clear technique to measure the completeness of data sets while responding to the next question. The only way to know if your AI is functioning well enough on the data it has been fed is to conduct a real-time clinical setting trial.
Moving on to the next question, he told us that at Synapsica, they do multiple rounds of annotation and take intermittent consensus to achieve an objective to use a true analogy as AI is like a dumb kid, and if one want that dumb kid to excel in trials where it is tested against multiple radiologists, then one would have to hand hold the AI to learn from multiple radiologists rather than a single radiologists. We compared our results to ground rules established by several technologists, which is one simple means of ensuring objectivity in the ground truths put into the AI system.
The method you use to compile your data or ground truth also contributes significantly to objectivity. When looking at the photos, picking out the observations is fairly objective. People can recognise the description by looking at the image, then use the description in conjunction with current medical criteria to come up with an interpretation. This also aids in the development of AI that is more understandable.
In addition to the answer to the next question, Dr. Cherian stated that the majority of AI businesses are preparing annotated photos, highlighting specific areas, and using masking technologies so that radiologists can see and comprehend the problem. They also provide radiologists with engagement, which they believe is vital as every AI outcome won’t be accurate all of the time. He went on to say that they think of AI as a junior radiologist in training who provides a report, which is then reviewed by senior radiologists who make modifications. We may learn where we are going wrong and what needs to be fixed by using feedback. The next question was answered by this.
Moving on to the following question: How does your deployment alter the care pathway, and can it be retrofitted? Yes, he replied, we can refit. While looking at the results of AI, radiologists should not switch to different systems because any or all of the efficiency gained from AI will be lost. In response to the question of changing the care pathway, he added that most AI solutions will improve the efficiency of the existing pathway and, in the next step, possibly change the overall clinical care pathway.
Moving on to the last question, Dr. Cherian explained that their AI system focuses on improving the efficiency of radiologists in reading and interpreting this type of exam, which is their main focus. They were able to achieve their goals of reducing the 15 minute time taken to 7 minutes for today’s cases by radiologists, and it involves automation of the manual processes that a radiologist will typically spend while reading and interpreting those types of exam. He also stressed the need to reduce burnout. A number of disorders may be made more sensitive with AI.
He mentioned that reaching a consensus is difficult, especially when it comes to radiologists who have been working in a certain way for a long time, and their work was done in a different way with AI. Now that they have resumed work and have worked for a long time, AI comes in and asks them to change their work behaviours, that is the most difficult part. The best part of AI is to have a documented proof of accuracy for the items, which will provide the professional the confidence in using the product. Apart from all this there is another issue to consider is for usability. With aforementioned words Dr. Cherian’s session came to a conclusion.
There are times when AI and physicians disagree, but just because one result differs from the other does not mean the AI is erroneous.
Mr. Gaur invited next and last panelist for day’s session Ms. Shraddha Mittal, Implementation Associate CARING Analytics platform(CARPL).

Ms. Mittal began by highlighting some of the hurdles that these AI solutions face on a regular basis when it comes to using them in real-world clinical workflows. She stated that CARPL is trying to become a single enabling player that provides healthcare providers global access to the greatest AI in medical imaging solutions while also ensuring that these AI solutions are seamlessly integrated into their day-to-day imaging workflow. She went on to say that they are in the process of deploying these solutions throughout their partner hospital sites around the world, resulting in CARPL being used in various locations on many continents. They are stationed at Thomas Jefferson University’s academic centres in the United States. They’re collaborating with Stanford’s Army Center, Mass General Hospitals, and other institutions in the area. She went on to say that they are highly active in Brazil at Albert Einstein Hospital and other imaging centres across the world. They are used in India at various hospitals and the Mahajan diagnostic chain.
Some of the issues, according to Shraddha initiates as healthcare providers are unaware of the existence of these AI solutions and their access alongwith with the knowledge to integrate the AI solutions into their daily workflow.
She described the lifecycle that CARPL conducts to effectively integrate AI solutions into hospital medical imaging operations with an attempt to add value to both AI developers and healthcare providers in this ecosystem. The IT infrastructure, she explained, is a key hurdle when it comes to deploying AI technologies in the healthcare ecosystem. As a result, they tend to shorten this period, and their relationship with AI partners is structured in such a way that they want them to concentrate on integrating their solutions. Then it’s up to them to spread that answer to as many hospitals as possible around the world. After that, they help with the integration of the AI technology into a hospital.
She mentioned that CARPL allows AI engineers to concentrate on designing more robust solutions as well as the deployment side of moving those solutions from the bench to the clinic. She informed us about the projects they are presently working on, as well as how CARPL can be used as a single interface to provide feedback from all around the world to AI developers in real time. When it comes to onboarding solutions, she stated that they are always on the lookout for high-accuracy solutions, ideally with FDA and CE licences. They’ve also assisted a few businesses in obtaining FDA approval. She finished by stating that CARPL is expanding into a variety of fields.
AI is a new way of thinking that needs to go, but that it should be remembered as a tool to assist medical professionals, not as a replacement for medicine, medical personnel, or doctors.
The club meeting then progressed to Q&A sessions.
Mr. Gaur and Dr. Singh wrapped up the meeting. Conclusive note by Mr. Sachin stated that AI in science is about knowing what we don’t know, not about money or productivity.
After that, Dr. V.K. Singh thanked the panellists and participants and elucidated that AI is a new way of thinking that needs to go, but that it should be remembered as a tool to assist medical professionals, not as a replacement for medicine, medical personnel, or doctors. He stated that he has faith in our people because of the vast amount of data we have because some of our states have more people than any other country. He thanked everyone for their participation in the meeting.
Composed by: “Clarion Smith Kodamanchili.”