Neural networks and deep learning have been employed in a range of translational research fields, such as image analysis, structural analysis, and sequence binding.
Affecting 15% of the global population, neurological illnesses are the most common cause of impairment, both mental and physical. Over the next 20 years, it is anticipated that the burden of chronic neurological ailments will only double due to the world’s ageing population. In light of this, maintaining universal access to neurological therapy will be very challenging. Alzheimer’s disease and Parkinson’s disease are the two neurodegenerative diseases that most frequently impact the elderly population.
One of the industries using wearable sensors, augmented and virtual reality, medical imaging, artificial intelligence, and other technologies most actively is the healthcare sector. Artificial intelligence is a fast-expanding field of research that tries to automate human intellect and recreate cognitive capacities using various approaches. It is becoming more and more relevant given the massive amount of huge data that is currently available.
A branch of artificial intelligence known as machine learning uses algorithms to identify patterns and extract significant features from massive datasets. Machine learning (ML) algorithms can be used to identify and forecast future outcomes once these patterns have been found and learned. In the medical field, machine learning can be used to data from several sources to help with tracking, diagnosis, and diagnostic-related tasks. ML systems, for example, can collect symptoms, register a patient’s response to treatment, and diagnose the severity of a disease in real-time remotely.
Like other medical specialties, neurology has benefited greatly from the integration of machine learning, particularly in the area of computer-aided detection, tracking, and treatment of symptoms related to neurodegenerative movement disorders.
Wearable technology and machine learning algorithms have been utilised to solve some of the difficulties related to neurological illness. ML has been used, for example, to follow and manage the progression of Parkinson Disease and to distinguish it from other conditions that appear similarly. The enhanced accuracy, dependability, accessibility, and efficiency of ML-integrated systems in clinical decision-making make them extremely promising for use in clinical practice. Moreover, ML has been applied to Alzheimer’s disease to monitor the illness’s course and serve as a source for differential diagnosis.
Instead of requiring manual interpretation by medical professionals, machine learning algorithms use computer-aided diagnosis to automatically identify and forecast the course of disease. This helps in clinical decision-making. A variety of methods are used to train machine learning models, such as ensemble model building, fresh model development, and transfer learning with pre-trained weights.
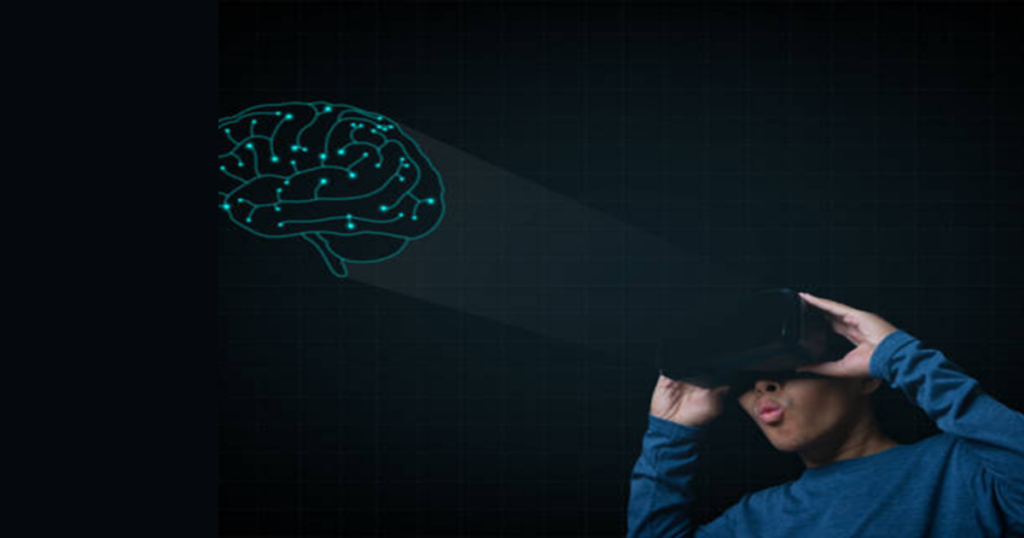
Machine Learning Contributions to The Computer-Aided Diagnosis of Neurodegenerative Diseases
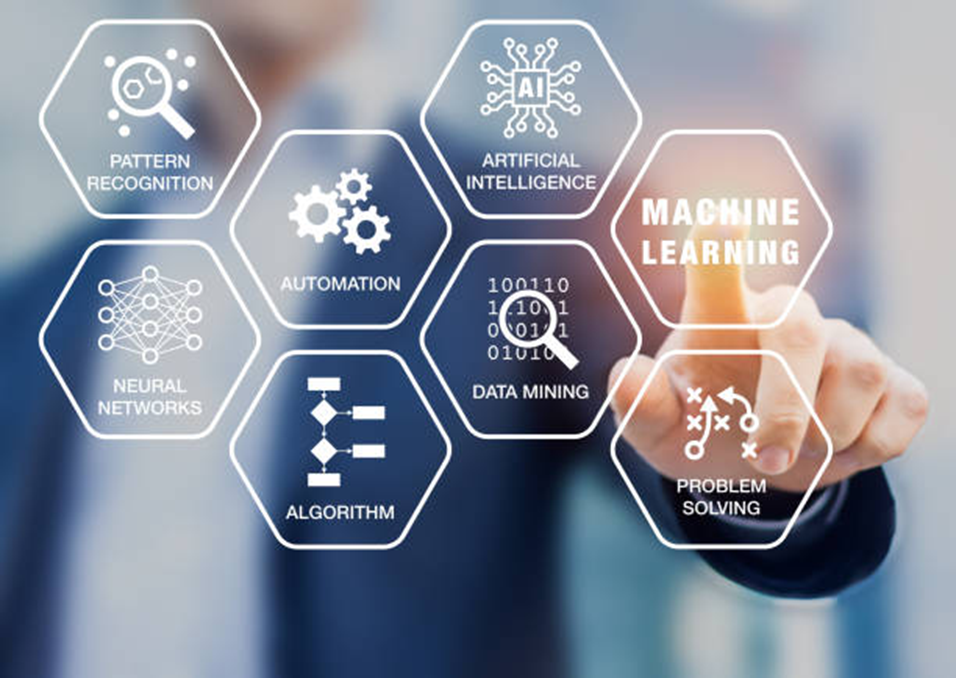
Parkinson’s and Alzheimer’s disease (AD) account for most cases of neurodegeneration. There are actual diseases like Parkinson’s disease (PD), motor neurone disease, Huntington’s disease, and many more; however, this article will focus on the two most prevalent ones, AD and PD. Deep learning is a new soft computing approach in machine learning that makes use of layered mathematical structures called neural networks. A hybrid model is a DL architecture that is combined with a more traditional ML architecture, such as a support vector machine (SVM) for classification. Neural networks and deep learning have been employed in a range of translational research fields, such as image analysis, structural analysis, and sequence binding. Because the higher-level characteristics of Deep Learning algorithms are more noise-resistant, they produce better outcomes.
Information is sent unidirectionally via hidden layers in an artificial neural network (ANN) from the input layer to the output layer. An extension of an artificial neural network (ANN) with several hidden layers is a deep neural network (DNN). Increasing the number of layers facilitates the learning and representation of intricate data patterns. Convolutional Neural Networks (CNNs) are specifically engineered for the processing of images and videos. Convolutional layers are used to automatically identify and extract feature spatial hierarchies from pictures. These look for local patterns, edges, and textures in the input image.
Convolutional neural networks (CNNs) and deep learning are tools that are used to find illness biomarkers and detect tiny brain changes, which allows for the early identification of disease.

Dysfunctions in working memory, planning, rule-finding, and set-shifting are collectively referred to as cognitive inertia. These deficits lead to indifferent conduct. Neurodegenerative illnesses of the Lewy body and Alzheimer’s disease are the main causes of cognitive loss.
A battery of computerised tests that measure cognitive stability indices focusing on the memory, attention, and response time domains has been developed in order to aid in the early detection of cognitive decline. Furthermore, by analysing the kinematic patterns of the head and hand during real-life tasks, the combination of virtual reality (VR) and artificial intelligence (AI) facilitated the continuous assessment of instrumental activities of daily life and led to the identification of behavioural measures capable of predicting nonverbal dysphoria (NDD).
Deep neural network speech analysis was successful in classifying AD patients into binary categories. Natural language processing (NLP) was used to extract rhythmic, acoustic, lexical, morpho-syntactic, and syntactic features from spontaneous speech transcriptions. This allowed for the early, multi-domain MCI to be distinguished from healthy controls, demonstrating both the method’s sensitivity to the progression of the disease and its ability to classify subtypes.
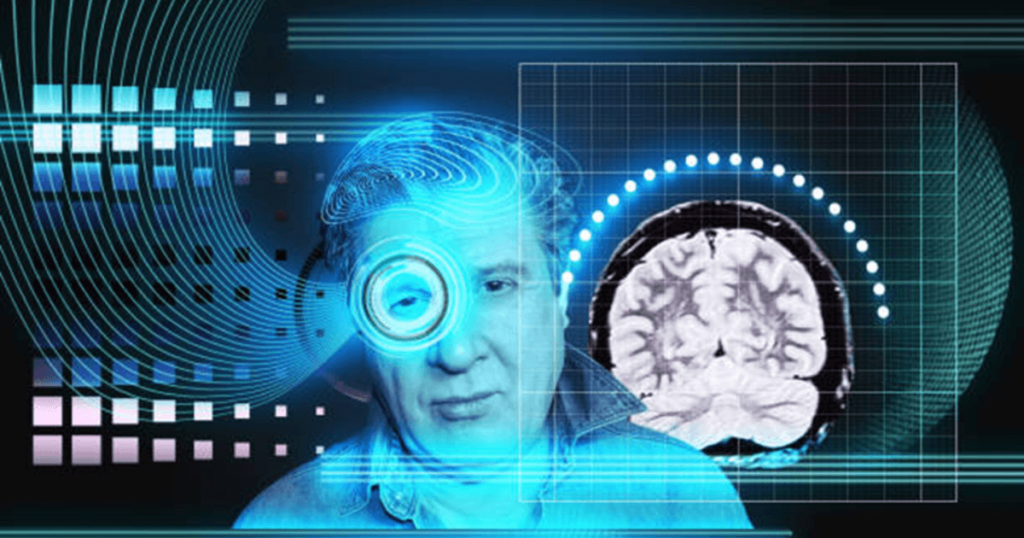
A comprehensive deep learning model was created, utilising a vision-based transformer, bidirectional encoder representation transformer, co-attention, multimodal shifting gate, and self-attention mechanism to understand the interplay between textual and spoken information.
Machine Learning Model (MLM)
Machine learning relies on the assumption that computer systems can learn from data. This method is intended to give software the capacity to learn from the collected data. For the “Therapeutic Robot and Artificial Intelligence in experimental Therapy” project, machine learning proved to be the most appropriate technique for making predictions on patients suffering from motor cognitive impairment. The purpose is to ascertain the degree of cognitive impairment in the patient and, in light of their individual objectives, provide the best rehabilitation strategy. A machine learning-based predictive statistical model was utilised to determine whether the patient’s cognitive impairment was present or absent.
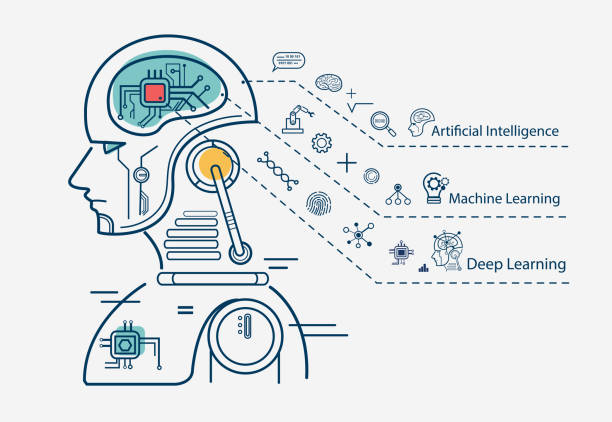
This neurodegenerative disorder is gaining more attention, maybe because there are no effective pharmaceutical therapies to halt the disease’s progression. Numerous research has backed the use of MLM based on neuroimaging biomarkers to better understand the aetiology of neurodegenerative illnesses and to aid in the differential diagnosis of AD. AI-driven algorithms are used to examine brain imaging data in medical image processing. Convolutional neural networks (CNNs) and deep learning are tools that are used to find illness biomarkers and detect tiny brain changes, which allows for the early identification of disease.
Furthermore, disease progression analysis and clinical outcome forecasting are conducted using AI’s predictive analytics capabilities. Through patient data analysis, AI models may detect patterns of sickness, calculate the rate of functional decline, and help physicians make informed decisions regarding therapy and care planning.
Algorithms can look at a range of data sources, such as genetic information, neuroimaging scans, and clinical assessments, to identify early signs and patterns suggestive of neurodegenerative disorders. Through early identification and an understanding of the minute changes that take place in the initial stages of the disease, numerical simulations aid in the development of computer models that depict the trajectory of the disease.
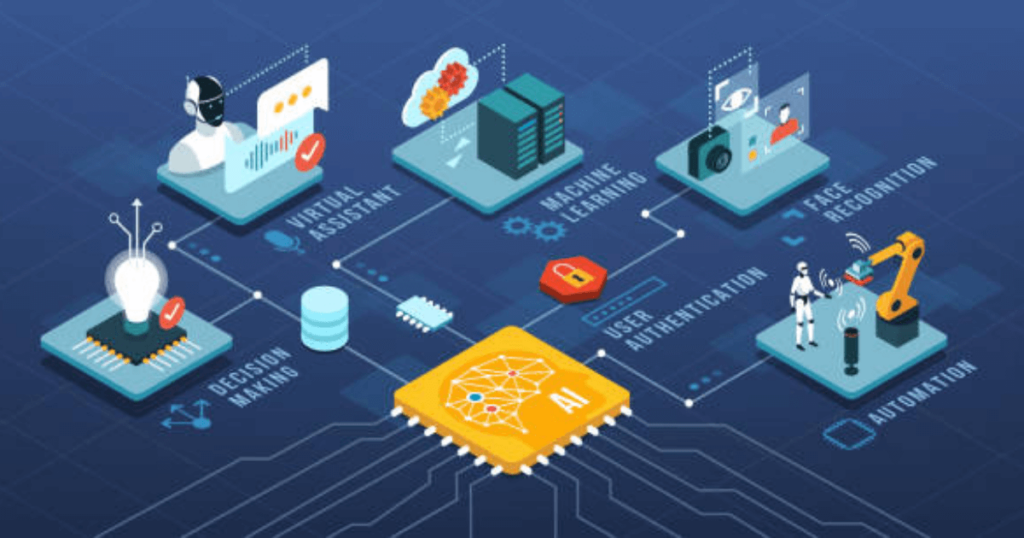
It will take some time before we can fully reap the benefits of artificial intelligence in the healthcare sector, as the technology is still in its infancy. Ahead of us lies a substantial amount of work, chief among which is the validation and optimisation of the existing models to produce more robust and long-lasting models.
“Composed by: ANUSHKA SAXENA a highly accomplished healthcare professional with background in physiotherapy, & now pursuing my Master’s degree in Hospital & healthcare management from Sharda University. Experienced in most widely used computer software, databases, healthcare terminologies, documents processing. Overall, a positive individual with a genuine interest in the well-being of patients & team mates with expertise in hygiene education.”